Tesla FSD Beta 10.13 Release Notes (2022.16.3.5)
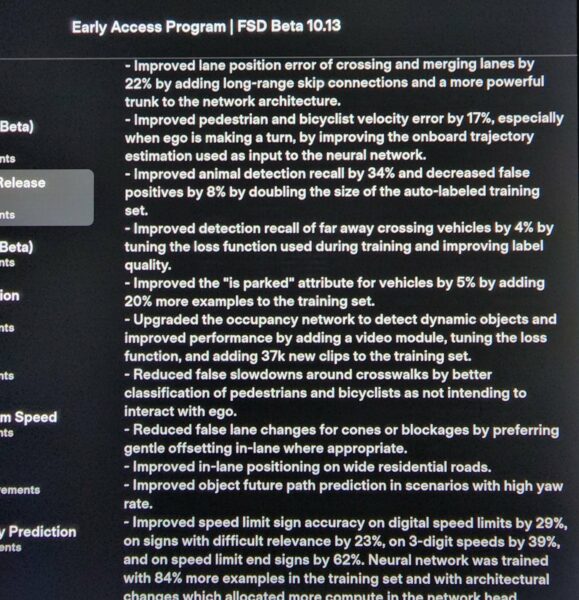
Photo: @WholeMarsBlog
After Tesla CEO Elon Musk said last week that Full Self-Driving (FSD) beta 10.13 would be coming this week, the new version of the driver assistance system is officially here.
Tesla’s FSD beta 10.13 has begun rolling out to internal testers and release notes have been shared by @WholeMarsBlog.
The release notes include a long list of updates, including what the automaker call improvements offering the “Chuck Cook” style of unprotected left turns — named after Cook’s several videos breaking down how unprotected left turns were handled by the system and how they could be improved.
Tesla FSD Beta for Right-Hand Drive Coming ‘Probably’ Later in 2022 https://t.co/uiJo1LXWT5
— TeslaNorth.com (@RealTeslaNorth) June 15, 2022
In addition, the release notes include improvements for creeping forward at turns for increased visibility, lane positioning on wide residential roads, merging, pedestrian and bicyclist recognition, animal detection and more.
Regarding far away vehicle recall improvements, Tesla wrote, “Improved detection recall of far away crossing vehicles by 4 percent by tuning the loss function used during training and improving label quality.”
Tesla’s FSD beta has continued to roll out to drivers above a 93 safety score.
FSD beta version 10.12 was released by Tesla in May, and upon release, Musk called it a “big one,” and said users should “expect some two steps forward, one step back situations.”
You can see Tesla’s complete list of FSD beta 10.13 release notes below:
FSD Beta v10.13 Release Notes
– Improved decision making for unprotected left turns using better estimation of ego’s interaction with other objects through the maneuver.
– Improved stopping pose while yielding for crossing objects at “Chuck Cook style” unprotected left turns by utilizing the median safety regions.
– Made speed profile more comfortable when creeping for visibility, to allow for smoother stops when protecting for potentially occluded objects.
– Enabled creeping for visibility at any intersection where objects might cross ego’s path, regardless of presence of traffic controls.
– Improved lane position error by 5% and lane recall by 12% with a
– Improved lane position error of crossing and merging lanes by 22% by adding long-range skip connections and a more powerful trunk to the network architecture.
– Improved pedestrian and bicyclist velocity error by 17%, especially when ego is making a turn, by improving the onboard trajectory estimation used as input to the neural network.
– Improved animal detection recall by 34% and decreased false positives by 8% by doubling the size of the auto-labeled training set.
– Improved detection recall of far away crossing vehicles by 4% by tuning the loss function used during training and improving label quality.
– Improved the “is parked” attribute for vehicles by 5% by adding 20% more examples to the training set.
– Upgraded the occupancy network to detect dynamic objects and improved performance by adding a video module, tuning the loss function, and adding 37k new clips to the training set.
– Reduced false slowdowns around crosswalks by better classification of pedestrians and bicyclists as not intending to interact with ego.
– Reduced false lane changes for cones or blockages by preferring gentle offsetting in-lane where appropriate.
– Improved in-lane positioning on wide residential roads.
– Improved object future path prediction in scenarios with high yaw rate.
– Improved speed limit sign accuracy on digital speed limits by 29%, on signs with difficult relevance by 23%, on 3-digit speeds by 39%, and on speed limit end signs by 62%. Neural network was trained with 84% more examples in the training set and with architectural changes which allocated more compute in the network head